Rita and Nik at the 2024 Machine Learning Summer School in Okinawa, Japan
We Rita González Márquez and Jan Niklas Böhm had the great opportunity to attend the Machine Learning Summer School at the Okinawa Institute for Science and Technology in the subtropical Okinawa island.
From the MLSS website: The machine learning summer school (MLSS) series was started in 2002 with the motivation to promulgate modern methods of statistical machine learning and inference. It was motivated by the observation that while many students are keen to learn about machine learning, and an increasing number of researchers want to apply machine learning methods to their research problems, only few machine learning courses are taught at universities. The Machine learning summer schools present topics which are at the core of modern Machine Learning, from fundamentals to state-of-the-art practice. The speakers are leading experts in their field who talk with enthusiasm about their subjects.
For two weeks we interacted with over 200 people from all over the world, learning about fundamental theoretical aspects of machine learning, as well as current topics; such as large language models (LLMs). Each day was structured into lectures separated by coffee breaks (and lunch), giving ample opportunity to talk to other attendees and exchange ideas. On every other day, the scientific program ended with a poster session, where every summer student got to present their work to eveyone else. Due to the sheer size, this almost felt like a regular conference, with a bustling crowd roaming through the rooms. Besides the scientific program, the organizers provided a few activities that gave a glimpse of the local culture of the island.
All in all, it was a well-rounded summer school and we were lucky to attend such a high quality seminar. And to top it all off, both of us got awards for our posters (as well as Kibidi Neocosmos, another student from Tübingen)!
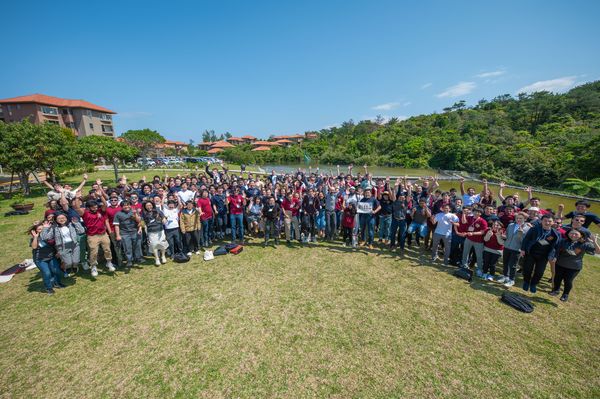
Share article
Author
Jan Niklas Böhm
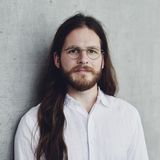