In the Department of Machine Learning, we develop models to improve decision making in clinical brain research.
About the Department of Machine Learning
We develop advanced machine learning and deep learning models to extract clinical information from neuroimaging, clinical, psychometric, smartphone, and omics data. We focus on challenges like explainability, robustness, confounding factors, causal inference, multimodal integration and adapting neural architectures (e.g., convolutional neural networks, transformers) to different data domains. Collaborating with clinicians, we apply these models to early detection of brain disorders (e.g., Alzheimer's, multiple sclerosis, depression), predict disease progression in chronic diseases (e.g., multiple sclerosis, stroke), and treatment responses in mental disorders (e.g., depression, anxiety, obsessive-compulsive disorder).
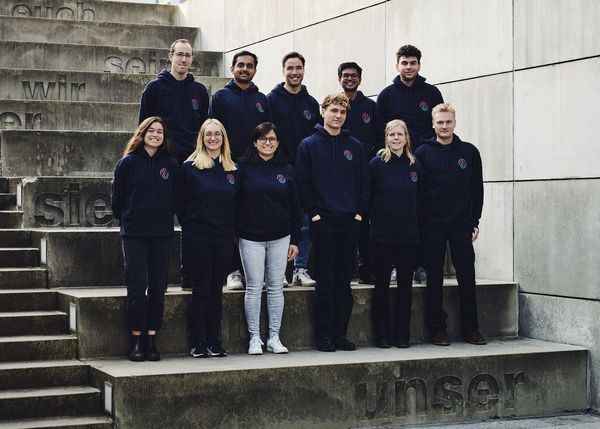
We are part of the Cluster of Excellence "Machine Learning - New Perspectives for Science" funded in the Excellence Initiative and the BMBF Competence Center for Machine Learning Tübingen AI Center. Our research is supported by the DFG, the BMBF, the Brain & Behavior Research Foundation and the Deutsche Multiple Sklerose Gesellschaft (DMSG). We support multiple interdisciplinary research consortia focusing on innovative methods that combine statistics and deep learning for biomedical data (RU 5363) or methodological advances in domain adaptation and workflow optimization (CRC 1404). Furthermore, we are involved in predictive modeling within specific clinical contexts, such as addiction (TRR 265) or the prediction of treatment response in depression and anxiety disorders using multimodal data (FOR 5187).
In collaboration with the BMBF-funded KI Campus, we have developed the highly successful podcast and interactive learning program Dr. med. KI. This program has achieved remarkable success, with over 20,000 downloads from more than 70 countries. It consists of three seasons that are continuously expanded and enhanced to provide an exceptional learning experience. Dr. med. KI is specifically designed for medical and computer science students and interested physicians, and it is open-source and CME-certified, making it suitable for continuing medical education.
Prof. Dr. Kerstin Ritter
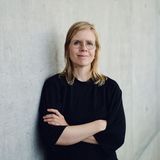