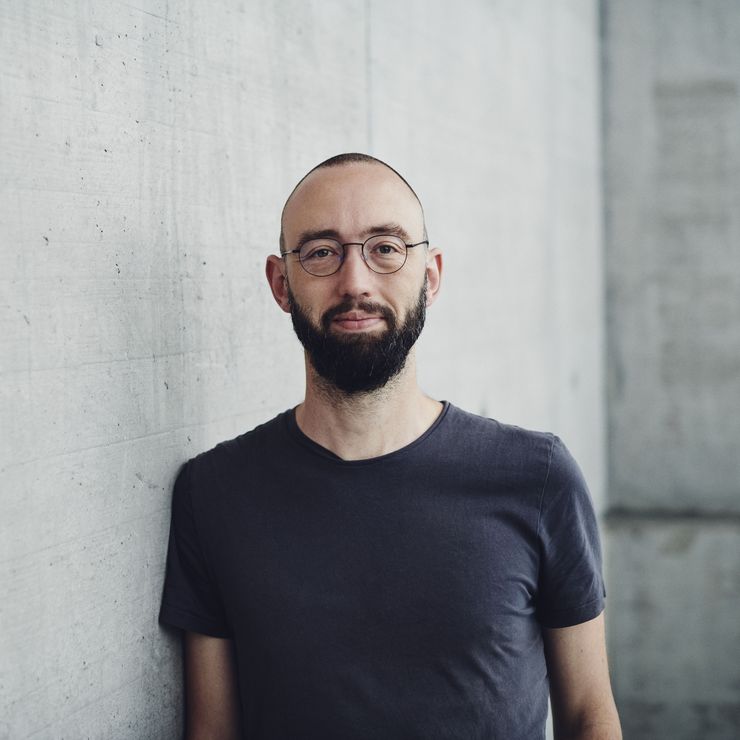
We apply machine learning algorithms to enable and accelerate discoveries in neuroscience and ophthalmology, which will ultimately allow us to diagnose diseases earlier and treat them better.
Prof. Dr. Philipp Berens
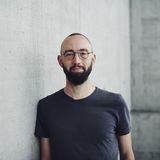
Prof. Dr. Philipp Berens is Full Professor of Data Science at the University of Tübingen and Director of the Hertie Institute for AI in Brain Health. Also, he is Speaker of the Excellence Cluster “Machine Learning – New Perspectives for Science” and is part of the core faculty of the Tübingen AI Center. His goal is to use machine learning to enable discoveries in basic and clinical neuroscience, with a focus on ophthalmology. He is interested in developing new algorithms whose output can be integrated into scientific or clinical workflows. His work has been recognized with a DFG Heisenberg Professorship, an ERC Starting Grant and the Bernstein Award of the German Ministry for Science and Education.
We apply machine learning algorithms to enable and accelerate discoveries in neuroscience and ophthalmology, which will ultimately allow us to diagnose diseases earlier and treat them better.
01
Beck, J., Bosch, N., Deistler, M., Kadhim, K.L., Macke, J. H., Hennig, P., Berens, P.
Diffusion Tempering Improves Parameter Estimation with Probabilistic Integrators for Ordinary Differential Equations
02
Zouridis, I. S., Schmors, L., Fischer, K. M., Berens, P., Preston-Ferrer, P., & Burgalossi, A.
Juxtacellular recordings from identified neurons in the mouse locus coeruleus
03
Wundram, A.M., Fischer, P., Wunderlich, S., Faber, H., Koch, L.M., Berens, P., Baumgartner C. F.
Leveraging Probabilistic Segmentation Models for Improved Glaucoma Diagnosis: A Clinical Pipeline Approach
04
Köhler, P., Fadugba, J., Berens, P., Koch, L.M.
Efficiently correcting patch-based segmentation errors to control image-level performance in retinal images
05
Yaïci, R., Cieplucha, M., Bock, R. et al
ChatGPT und die deutsche Facharztprüfung für Augenheilkunde: eine Evaluierung
06
Koch, L.M., Baumgartner, C.F. & Berens, P
Distribution shift detection for the postmarket surveillance of medical AI algorithms: a retrospective simulation study
07
Ayhan, M. S., Neubauer, J., Uzel, M. M., Gelisken, F., & Berens, P.
Interpretable detection of epiretinal membrane from optical coherence tomography with deep neural networks.
08
González-Márquez, R., Schmidt, L., Schmidt, B. M., Berens, P., & Kobak, D.
The landscape of biomedical research
09
Grote, T., Berens, P.
A paradigm shift?—On the ethics of medical large language models
10
Ayhan, M. S., Faber, H., Kühlewein, L., Inhoffen, W., Aliyeva, G., Ziemssen, F., & Berens, P.
Multitask Learning for Activity Detection in Neovascular Age-Related Macular Degeneration
11
Djoumessi, K. R. D., Ilanchezian, I., Kühlewein, L., Faber, H., Baumgartner, C. F., Bah, B., Berens, P. & Koch, L. M.
Sparse Activations for Interpretable Disease Grading
12
Grote, T., & Berens, P
Uncertainty, evidence, and the integration of machine learning into medical practice
13
Congiu, M., Mondoloni, S., Zouridis, I. S., Schmors, L., Lecca, S., Lalive, A. L., Ginggen, K., Deng, F., Berens, P., Paolicelli, R. C., Li, Y., Burgalossi, A. & Mameli, M.
Plasticity of neuronal dynamics in the lateral habenula for cue-punishment associative learning
14
Franke, K., Cai, C., Ponder, K., Fu, J., Sokoloski, S., Berens, P., & Tolias, A. S.
Asymmetric distribution of color-opponent response types across mouse visual cortex supports superior color vision in the sky.
15
Böhm, J. N., Berens, P., & Kobak, D.
Unsupervised visualization of image datasets using contrastive learning
16
Janschewski, J., Käppler, C., & Berens, P.
School predictors of mental health problems in children and adolescents based on a survey of students in hospital and regular schools
17
Boreiko, V., Augustin, M., Croce, F., Berens, P., & Hein, M.
Sparse visual counterfactual explanations in image space
18
Koch, L. M., Schürch, C. M., Gretton, A., & Berens, P.
Hidden in plain sight: Subgroup shifts escape ood detection
19
Beck, J., Deistler, M., Bernaerts, Y., Macke, J. H., & Berens, P
Efficient identification of informative features in simulation-based inference
20
21
Böhm, J. N., Berens, P., & Kobak, D.
Attraction-repulsion spectrum in neighbor embeddings
22
Blum, C., Baur, D., Achauer, L. C., Berens, P., [...], Huang, Z., [...] , Macke, J.H., [...] & Ziemann, U.
Personalized neurorehabilitative precision medicine: from data to therapies (MWKNeuroReha)–a multi-centre prospective observational clinical trial to predict long-term outcome of patients with acute motor stroke
23
Strauss, S., Korympidou, M. M., Ran, Y., Franke, K., Schubert, T., Baden, T., Berens, P., Euler, T. & Vlasits, A. L
Center-surround interactions underlie bipolar cell motion sensitivity in the mouse retina
24
Boreiko, V., Ilanchezian, I., Ayhan, M. S., Müller, S., Koch, L. M., Faber, H., Berens, P. & Hein, M.
Visual explanations for the detection of diabetic retinopathy from retinal fundus images
25
Oesterle, J., Krämer, N., Hennig, P., & Berens, P.
Probabilistic solvers enable a straight-forward exploration of numerical uncertainty in neuroscience models
26
Faber, H., Berens, P., & Rohrbach, J. M.
Ocular changes as a diagnostic tool for malaria
27
Ayhan, M. S., Kümmerle, L. B., Kühlewein, L., Inhoffen, W., Aliyeva, G., Ziemssen, F., & Berens, P.
Clinical validation of saliency maps for understanding deep neural networks in ophthalmology
28
Behrens, C., Yadav, S. C., Korympidou, M. M., Zhang, Y., Haverkamp, S., Berens, P. & Schubert, T.
Retinal horizontal cells use different synaptic sites for global feedforward and local feedback signaling.
29
Gonschorek, D., Höfling, L., Szatko, K. P., Franke, K., Schubert, T., Dunn, B., Berens, P. ... & Euler, T.
Removing inter-experimental variability from functional data in systems neuroscience.
30
BRAIN Initiative Cell Census Network (BICCN)
A multimodal cell census and atlas of the mammalian primary motor cortex
31
Yoshimatsu, T., Bartel, P., Schröder, C., Janiak, F. K., St-Pierre, F., Berens, P., & Baden, T.
Ancestral circuits for vertebrate color vision emerge at the first retinal synapse
32
Scala, F., Kobak, D., Bernabucci, M., Bernaerts, Y., Cadwell, C. R., Castro, J. R., [...], Berens, P. & Tolias, A. S.
Phenotypic variation of transcriptomic cell types in mouse motor cortex
33
Ilanchezian, I., Kobak, D., Faber, H., Ziemssen, F., Berens, P., & Ayhan, M. S
Interpretable gender classification from retinal fundus images using BagNets.
34
Lause, J., Berens, P., & Kobak, D.
Analytic Pearson residuals for normalization of single-cell RNA-seq UMI data.
35
Huang, Z., Ran, Y., Oesterle, Y., Euler, T., Berens, P.
Estimating smooth and sparse neural receptive fields with a flexible spline basis
36
Kobak, D., Bernaerts, Y., Weis, M. A., Scala, F., Tolias, A. S., & Berens, P.
Sparse reduced-rank regression for exploratory visualisation of paired multivariate data
37
38
Schroeder, C., Oesterle, J., Berens, P., Yoshimatsu, T., & Baden, T.
Distinct synaptic transfer functions in same-type photoreceptors.
39
Baden, T., Euler, T., & Berens, P.
Understanding the retinal basis of vision across species.
40
Schröder, C., Klindt, D., Strauss, S., Franke, K., Bethge, M., Euler, T., & Berens, P.
System identification with biophysical constraints: A circuit model of the inner retina.
41
Oesterle, J., Behrens, C., Schröder, C., Hermann, T., Euler, T., Franke, K., ... & Berens, P.
Bayesian inference for biophysical neuron models enables stimulus optimization for retinal neuroprosthetics
42
Pfau, M., Walther, G., von der Emde, L., Berens, P., Faes, L., Fleckenstein, M., ... & Holz, F. G.
Artificial intelligence in ophthalmology: Guidelines for physicians for the critical evaluation of studies.
43
Ayhan, M. S., Kühlewein, L., Aliyeva, G., Inhoffen, W., Ziemssen, F., & Berens, P.
Expert-validated estimation of diagnostic uncertainty for deep neural networks in diabetic retinopathy detection.
44
Laturnus, S., von Daranyi, A., Huang, Z., & Berens, P.
MorphoPy: A python package for feature extraction of neural morphologies.
45
Yoshimatsu, T., Schröder, C., Nevala, N. E., Berens, P., & Baden, T.
Fovea-like photoreceptor specializations underlie single UV cone driven prey-capture behavior in zebrafish.
46
Szatko, K. P., Korympidou, M. M., Ran, Y., Berens, P., Dalkara, D., Schubert, T., ... & Franke, K.
Neural circuits in the mouse retina support color vision in the upper visual field.
47
Meding, K., Bruijns, S. A., Schölkopf, B., Berens, P., & Wichmann, F. A.
Phenomenal causality and sensory realism.
48
Laturnus, S., Kobak, D., & Berens, P.
A systematic evaluation of interneuron morphology representations for cell type discrimination.
49
Ran, Y., Huang, Z., Baden, T., Schubert, T., Baayen, H., Berens, P., ... & Euler, T.
Type-specific dendritic integration in mouse retinal ganglion cells.
50
Höfling, L., Oesterle, J., Berens, P., & Zeck, G
Probing and predicting ganglion cell responses to smooth electrical stimulation in healthy and blind mouse retina.
51
Zhao, Z., Klindt, D. A., Maia Chagas, A., Szatko, K. P., Rogerson, L., Protti, D. A., ... & Euler, T.
The temporal structure of the inner retina at a single glance.
52
Power, M. J., Rogerson, L. E., Schubert, T., Berens, P., Euler, T., & Paquet‐Durand, F.
Systematic spatiotemporal mapping reveals divergent cell death pathways in three mouse models of hereditary retinal degeneration.
53
Cadwell, C. R., Scala, F., Fahey, P. G., Kobak, D., Mulherkar, S., Sinz, F. H., ... & Tolias, A. S.
Cell type composition and circuit organization of clonally related excitatory neurons in the juvenile mouse neocortex.
54
Berens, P., Waldstein, S. M., Ayhan, M. S., Kuemmerle, L., Agostini, H., Stahl, A., & Ziemssen, F.
Potential of methods of artificial intelligence for quality assurance.
55
Grote, T., & Berens, P.
On the ethics of algorithmic decision-making in healthcare.
56
Schröder, C., James, B., Lagnado, L., & Berens, P.
Approximate bayesian inference for a mechanistic model of vesicle release at a ribbon synapse.
57
Kobak, D., & Berens, P.
The art of using t-SNE for single-cell transcriptomics.
58
Kobak, D., Linderman, G., Steinerberger, S., Kluger, Y., & Berens, P.
Heavy-tailed kernels reveal a finer cluster structure in t-SNE visualisations.
59
Scala, F., Kobak, D., Shan, S., Bernaerts, Y., Laturnus, S., Cadwell, C. R., ... Berens, P., ... & Tolias, A. S
Layer 4 of mouse neocortex differs in cell types and circuit organization between sensory areas
60
Rogerson, L. E., Zhao, Z., Franke, K., Euler, T., & Berens, P.
Bayesian hypothesis testing and experimental design for two-photon imaging data.
61
Rosón, M. R., Bauer, Y., Kotkat, A. H., Berens, P., Euler, T., & Busse, L.
Mouse dLGN receives functional input from a diverse population of retinal ganglion cells with limited convergence.
62
Bellet, M. E., Bellet, J., Nienborg, H., Hafed, Z. M., & Berens, P
Human-level saccade detection performance using deep neural networks.
63
Berens, P., & Ayhan, M. S.
Proprietary data formats block health research.
64
Dhande, O. S., Stafford, B. K., Franke, K., El-Danaf, R., Percival, K. A., Phan, A. H., ..., Berens, P., ... & Huberman, A. D