Data Science / Representation Learning and Data Visualization
We develop machine learning tools for visualizing high-dimensional data such as single cell transcriptomics, clinical images, or scientific texts.
Representation Learning and Data Visualization
We are interested in dimensionality reduction and data visualization of single-cell transcriptomic and multi-omic data, as well as scientifically relevant image and text datasets. We believe that low-dimensional data visualization is a powerful tool that enables data exploration, helps to generate hypotheses, and allows researchers to spot inconsistencies in their data. To this end, we develop manifold and contrastive learning methods, that yield biologically or scientifically meaningful two-dimensional representations of single-cell data or medical images. We also develop methods for dimensionality reduction of multi-omic datasets, such as Patch-seq data combining transcriptomic and electrophysiological recordings and use large-language models for embedding scientific texts.
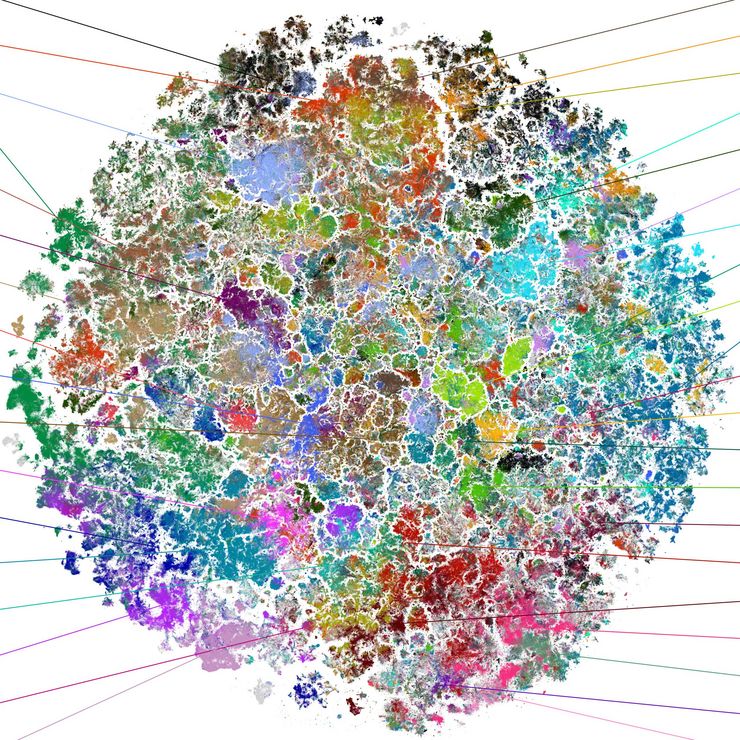