Data Science / Neuronal Modeling
We gain insights into the healthy and diseased nervous system by developing and using computational models.
Neuronal Modeling
We develop new machine learning algorithms for analyzing neurons and neural circuits based on large scale and multimodal neural data, including electrophysiology, two-photon imaging, high-resolution anatomical reconstructions, and single-cell transcriptomes. We are interested in developing a new class of tractable models between traditional biophysically realistic models and purely statistical ones. For learning the model parameters from data, we resort to the latest developments in machine learning such as likelihood-free inference or probabilistic numerics. We believe that such hybrid models will help us reach an integrative understanding of retinal circuits across scales, and help integrating complex datasets from disparate sources. Also, we are interested in using machine learning approaches in sensory ecology.
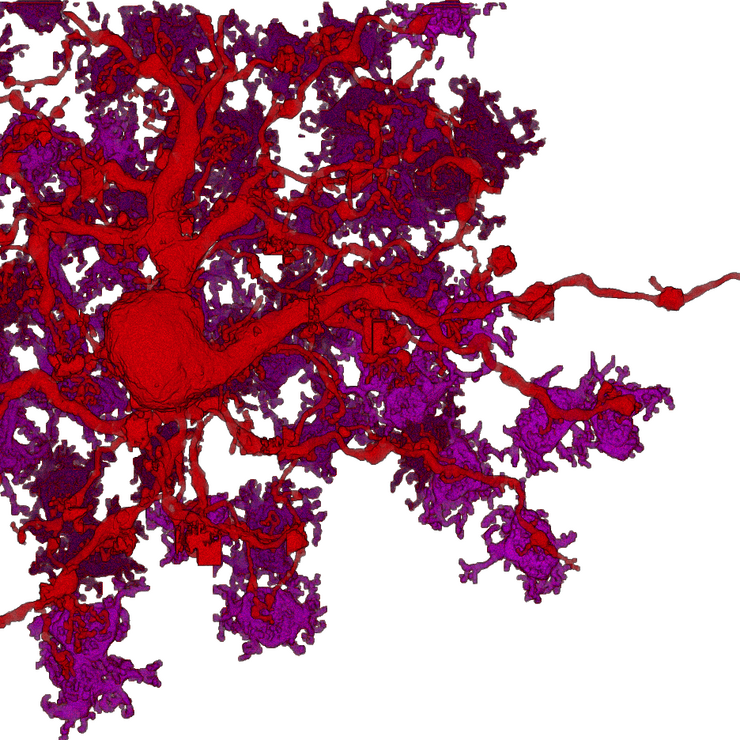