Widening Perspectives at MIDL 2024 in Paris
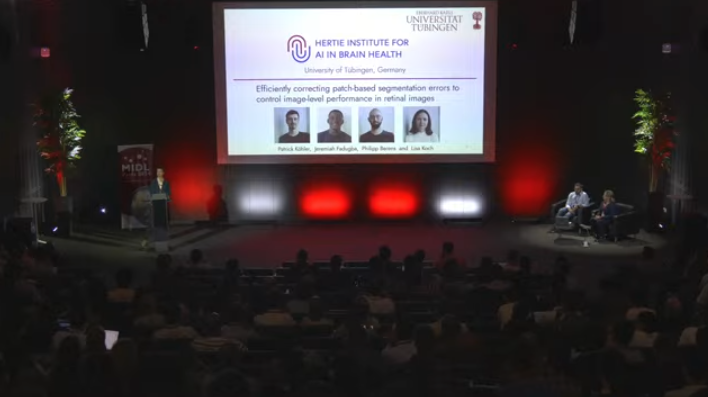
My presentation is scheduled for the very first slot of the conference. I’ve never spoken to multiple hundred people before, which makes me a little bit nervous during my train ride to arguably one of the most beautiful cities in Europe. Tinges of wow surface on every corner between pompous buildings on my way to the campus. The conference venue reminds me much more of a modern cinema than a lecture hall. On top of that it’s located right between the Seine river and a botanical garden, making it perfect for some easy strolls in the lunch break.
Bright lights are directed at me when I start summarizing over one year of brainstorming, programming and discussing into a thirteen minute talk with ten slides. My pupils are widening as I look up from the presenter’s desk and gaze across the crowd. Having the attention of so many people feels empowering now and I enjoy my talk. During the poster session, many people come to figure out mathematical details or give thoughtful impulses for conceptual extensions and the adoption of our method into clinical practice. I feel relieved and happy that we get so much good feedback and that I have condensed our work successfully into a thirteen minute talk.
The conference covers a wide range of topics in terms of methodology but always revolves around the application of deep learning for medical image analysis. What sets this event apart from other conferences? The papers highlight the intricacies of applying Machine Learning models in the clinical practice, which raises problems that are often not considered in the major machine learning conferences.
More than anything else, browsing the other posters and discussing their lines of work, substantiates my optimism that work from the academic world already contributes to improving healthcare in many ways. Seeing so many different projects right next to each other widens my perspective immensely. The tools that we build are not only going to be applied in the domains for which we develop them but have the potential to improve multiple medical disciplines.
One of the keynote talks, given by Gael Varoquaux, stood out for me. In essence, he reminded if not admonished us to tackle the basic questions, such as Explainability, Uncertainty Quantification and Robust Generalization again and again from different angles until we have satisfactory answers. We should not focus too much on improving architectures and pushing benchmarks but ensure that our models meet the needs of the clinicians and withstand the challenges of real world application.
Share article
Author
Patrick Köhler
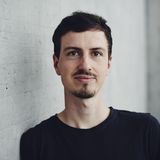