Attending the 2024 International Conference on Learning Representations (ICLR) in Vienna
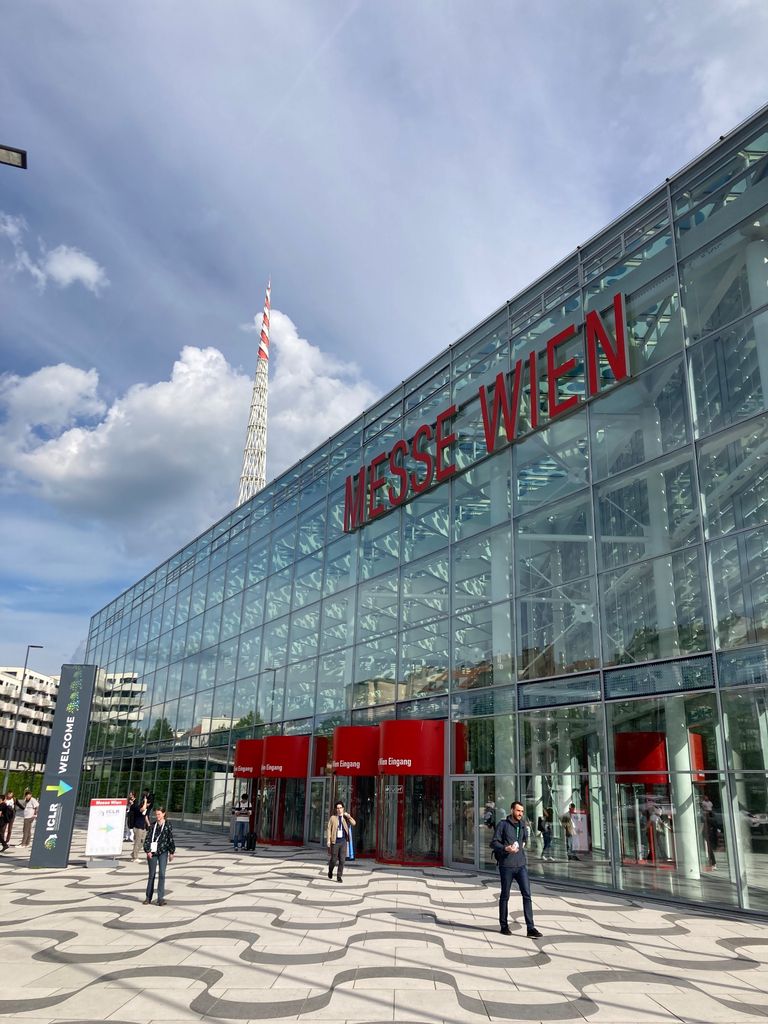
The International Conference on Learning Representations (ICLR) is one of the largest conferences in the field of machine learning, and thus a highlight in the calendar for researchers every year. This year, I had the pleasure of attending the conference, which took place in Vienna from the 7th to the 11th of May. It was an enriching experience filled with learning and networking.
The conference features a series of keynotes, poster sessions, and orals the first 4 days, along with other events. The last day is dedicated to the workshops, where more specific research topics are covered by the different workshops, which run in parallel.
One of the aspects that I personally find most exciting of attending the conference are the poster sessions. These sessions are very intense and very rewarding, since one has the opportunity of getting and overview of more than 200 papers being presented at the same time, and select and focus on the ones of ones interest. Walking through the aisles, I encountered many works that were highly related and relevant to my research. The interactive nature of the poster sessions is what makes it special – one can engage with the presenters, ask questions, and even brainstorm potential collaborations.
The workshops and keynote speeches were very interesting as well. One workshop that stood out was “How far are we from AGI”, where several experts in the field – including Joshua Bengio, Dawn Song, and Ge Liu – held a remarkable panel discussion on the development of artificial general intelligence (AGI) and AI safety. The keynotes, particularly the one by Raia Hadsell, were inspiring and provided a glimpse into the future directions of machine learning. Her metaphor of AI models as multitudes or monoliths and her opinions on which modality can be the strongest base ground for future AI models were very interesting.
A personal highlight for me was presenting a poster on our recent paper in the workshop “Data-centric machine learning research”. Our paper focused on introducing a dataset of abstracts of machine learning articles – the ICLR dataset – which we use to study the evolution of the field of ML. Additionally, we propose to use the dataset as a benchmark for evaluating quality of large language models embeddings. Our paper is now publicly available and can be found on https://arxiv.org/abs/2404.08403 . The audience’s engagement was encouraging; their questions and feedback provided valuable insights and opened up new perspectives on our work.
ICLR offered abundant networking opportunities: from informal chats during coffee breaks to more structured networking events. One of the Socials I enjoyed the most was “Women in Machine Learning”, where women and non-binary individuals are able to network and discuss aspects related to ML research and the difficulties we face as women, and learn from the experiences from others. It was accompanied by a panel discussion, where four women with senior positions in industry and academia shared their views on many different topics related to their career path, personal development, and the evolution of machine learning research. With only 18% of the conference’s attendees being women, this type of events are encouraging and necessary to highlight and combat the gender imbalance in machine learning research.
Attending ICLR was all in all an immensely rewarding experience. The conference not only increased my knowledge of the latest research in the field, but also sparked new ideas and inspiration for my own research. Attending a conference such as this is something I would recommend to any PhD student, and I am very grateful I had the opportunity to do so.
Share article
Author
Rita González Márquez
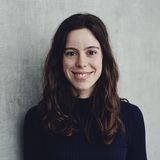