A brief summary of ICML 2024
It was a warm Saturday morning at the end of July, when my train left the station in Tübingen. It was headed for Vienna, and I for the international conference of machine learning, to see how good LLMs had become at predicting the next token, to find out about the latest trends in "AI for Science" and to present my most recent piece of work in probabilistic numerics.
On Sunday the conference officially started and after collecting my credentials, I took the first tour of the premises and met up with colleagues from Tuebingen, to take a peak into the first tutorial and to dive into the Donau afterwards (mackelab).
Monday was another day full of tutorials, the most memorable and insightful of which was about the Physics of Language Models by Zeyuan Allen-Zhu, who studied the behaviour of LLMs in highly controlled environment, do derive intuitive governing laws for them. The day ended with the official welcome reception.
Tuesday was the start of the main conference, which started with a great keynote by Soumith Chintala on Unapologetically Open Science -- the complexity and challenges of making openness win!, in which he gave an opinionated view on the benefits of open source science. Straight after, the first round of orals – one one time-series – took place and followed by the first poster session of the conference, where me and Michael Deistler were already scheduled to present our paper Diffusion Tempering Improves Parameter Estimation with Probabilistic Integrators for Ordinary Differential Equations. There was a lot of interest in our work and the 90 minutes that were allocated went by in a blink. We had tons of great discussions, some of which we were able to follow up on in the subsequent lunch and coffee breaks. The 1st poster session was followed by a really great oral from Minyoung Huh and Brian Cheung on "The Platonic Representation Hypothesis" and then the 2nd poster session of the day. Unsuprisingly the poster session was dominated by LLM and diffusion models, but I had a few posters here and there that I found interesting.
Wednesday was again a mixture of orals and posters, although none of them I found really noteworthy. Javier Duarte however gave an interesting keynote on Machine Learning Opportunities for the Next Generation of Particle Physics and the challenges of replacing the often old school data pipelines with modern machine learning algorithms.
Thursday was the last day of the main conference and again had a few neat orals and posters that I stumbled across with the help of the scholar inbox ,paper recommender for the conference. Highlights of day 3 included the keynote by Lucilla Sioli on the View of AI from the European Commission and Manuel Glöcklers oral on his incredible paper All-in-one simulation-based inference.
Friday and Saturday were dedicated to workshops. There were a few very interesting ones that I weaved in and out of during the day, namely Differentiable Almost Everything: Differentiable Relaxations, Algorithms, Operators, and Simulators, the AI for Science: Scaling in AI for Scientific Discovery and the ML for Life and Material Science: From Theory to Industry Applications. While the AI for science field seems to be dominated by molecules, proteins and material generation using diffusion models, there were a few cool papers on inverse problem solving using diffusion models and the folks from the BrainPy library presented their package.
Overall I am really happy and thankful that I got to attend ICML this year. It was a great opportunity to meet with colleagues, discuss ideas, learn about the current state of the field and to just experience the vibrant ML community.
Share article
Author
Jonas Beck
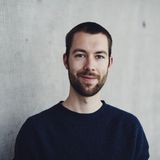